METHODS Flashcards
Method definitions, theories, assumptions, limitations, procedures, tips and tricks, etc.
Demand Characteristics
When the participant changes their true response due to how they believe the experimenter wants it to go.
Expectancy Effects
When the experimenter’s biases cause them (consciously or unconsciously) to sway the experiment to match how they expect it to go.
Regression Discontinuity Design
A type of quasi-experimental design where you create an artificial control group by looking at people who fall close to a particular cutoff score, given that the cutoff score elicits the treatment. (ie, study outcomes of students with a grade of 79% compared to those at 80% who received a scholarship)
What are the 5 types of Quasi-experimental design?
Single group without a control Multigroup without a pretest Multigroup with control and pretest Time series designs Regression discontinuity
What are the 3 ingredients necessary for a randomized experimental design?
Random assignment Manipulates the independent variables Measurement of dependent variables
Grounded Theory of Causal Generalization
Shadish, Cook, and Campbell, 2002 1. Surface Similarity 2. Ruling Out Irrelevancies 3. Making Discriminations 4. Interpolation & Extrapolation 5. Causal Explanation
Multicollinearity
When there is a very high (or even perfect) correlation between independent variables. It’s a good indication that the data is not reliable. (Edwards 2008)
Construct Validity
the degree of correspondence between the constructs (i.e., latent traits) referenced by a researcher and their empirical realization (i.e., operationalization/measure); in other words, is it measuring what it claims to be measuring?(Stone-Romero, 2011)
Bias
systematic variance in an operational deginnition that is unrelated to the focal construct (Stone-Romero, 2011)
Unreliability
nonsystematic (random) variance in an operational definition (Stone-Romero, 2011)
Deficienct
when the constructs empirical realization (operationalization/measure) does not fully capture the essence of the construct (Stone-Romero, 2011)
Special Purpose vs. Non-Special Purpose Settings
More appropriate than a field vs lab distinction SP- created for the specific purpose of doing research (e.g., university labs, simulated work settings in an org); designed to allow for the effective (unconfounded) manipulation of one or more independent variables NSP- created for the purposes other than reserach (e.g., organizations, classrooms, churches, etc. )
Probability sampling
workers are selected from a population (with N members) in a way that ensures that the probability of selection a sample of a given sze (e.g., n=10) is equal to the probbilty of selection any other sample of the same size… I.E., all people in the population have an equal chance at being selected to participate– rarely occurs in IO for many obvious reasons
Startified random sampling
a researcher specifies strate of employees within an org (e.g., managerial, nonmanagerial) and then randomly select a specific number of workers from each stratum of interest
multistage cluster sampling
a researcher a) selects a random sample of units in one or moer clusters (e.g., private vs. public-sector, profit vs. non profit) and then b) randomly selects clusters of orgs from each larger cluster
nonprobabilty sampling
uses non random strategies for selecting sample members from a target population e.g., convenience sampling, purposive sampling of heterogeneous instances, systematic sampling, quota sampling
convenience sampling
selecting a nonrandom sample based on their availability to to participate in a study (i.e., intro to psych students)
purposive sampling of heterogeneous instances
selecting a nonrandom sample of members on the basis that the researcher believes that they are diverse in terms of characteristics that might influence a causal relation between variables (Shadish et al., 2002)
systematic sampling
select sample members in a methodical way from lists of assumed population members (e.g., every 10th person in the SIOP directory)
quota sampling
selecting specific numbers of sample members of different types to produce a sample that is roughly representative of the target population
Critiques of IO rigorous reserach
a) has little to no practical utility b) does not inspire confidence in the work of scientiests c) distorts important aspects of reality in orgs d) fails to consider phenomenon that are not readily measurable e) has low releance to the criteria that are important to practitioners f) doesn’t offer much more than common sense/common knowledge g) it is artificial/ irreleant/trivial j) has low operational validity (aka variables that can’t be controlled by practitioners realistically or ethically k) focuses on “proving” the truth of hypotheses and not problem solving l) untimely- deals with issues that change more rapidly than the knowledge it produces m) involves participants who are not representative of the populations to which generalizations are to be made (Stone-Romero, 2011; Thomas & Tymon, 1982)
Critique of NSP Causal Claims
- harder to control extraneous and confounding variables even using random experiments or quasi-experiments - the claim that there is more mundane realism and thus more external validity doesn’t always hold up - typically involce nonrepresentative samples of subjects, settings, and operational definitions of manipulations/measures - Locke (1986) showed evidence that typically relations found in SP settins hold up in NSP settings.
Mundane Realism
Is it consistent with the every day realities (e.g., adding health benefits, vacation time, etc. to simulated orgs even if it is unrelated to the study); if a study lacks mundance realism, an effect found in SP settings may not generalize to NSP settings (Stone-Romero, 2011)
Experimental Realism
the manipulations/measures are operationally defined such that they have a suffidient impact on study participants, it is noticable, it is legitimate enough to show an effect; if it lacks this, models may fail to show support for causal connections between variables, even if there is one i.e., why manipulation checks are important (Stone-Romero, 2011)
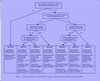

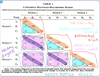





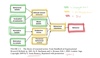







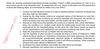

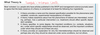
